All published articles of this journal are available on ScienceDirect.
The Role of Molecular Modeling and Bioinformatics in Treating a Pandemic Disease: The Case of COVID-19
Abstract
The COVID-19 pandemic first appeared in Wuhan, China, in December 2019 in a cluster of pneumonia patients. The causative agent was found to be SARS-CoV-2. Here, we are summarizing current treatment strategies and highlighting the role of bioinformatics, molecular modeling, and structural biology during the COVID-19 pandemic. There are different pharmacological treatments, mostly repurposed drugs, employed for the treatment of COVID-19, including antiviral drugs, corticosteroids, biologic drugs, antibiotics, antifungal agents, and anticoagulants. Some immune-based therapies are also under evaluation, including convalescent plasma, IL-1, IL-6 inhibitors, and interferons. Different bioinformatics networks are established to provide information about the structure, transcriptome, and pathogenicity of the virus. The genotyping analysis for SARS-CoV-2 is also useful in identifying different mutations, SNPs, and conservative domains along the viral genome. Cryo-EM and X-ray diffraction had a crucial role in determining the structure of viral proteins such as spike (S) protein, main protease, and RdRp. NMR had a minor role and determining the structure of nucleocapsid (N) protein only. Several docking studies were performed to predict the interaction of certain FDA-approved drugs with known efficacy and toxicity, while others used natural products. Among different study types, in silico drug prediction and repurposing have the lowest risk with less off-target results. Therefore, bioinformatics and in silico studies have an important role during pandemics in providing information about viral structure and function and predicting potential treatments.
1. INTRODUCTION
1.1. The Appearance, Structure, and Origin of SARS-CoV-2
The world is recently facing Coronavirus Disease (COVID-19) that threatens public health everywhere. COVID-19 first appeared in Wuhan, China December 2019 in a cluster of pneumonia patients [1]. It was pronounced a global pandemic disease by the World Health Organization (WHO) on March 11th, 2020. The causative agent of COVID-19 belongs to RNA β- Coronaviruses. It has a 79.5% similarity with the genome of the Severe Acute Respiratory Syndrome Coronavirus (SARS-CoV) that caused an outbreak during 2002-2003 [2]. The new virus also utilizes the angiotensin-converting enzyme 2 (ACE2) receptor for human cell entry [2]. WHO named the new virus SARS-CoV-2. It is an enveloped, positive-sense RNA, a single-stranded virus with a non-segmented genome. The viral genome of SARS-CoV-2 is very similar to that of other coronaviruses. It contains different numbers of open reading frames (ORFs), with two-thirds of the viral genome located in the first one (ORF1a/b) encoding 16 non-structural proteins (Nsps). The rest of the ORFs encodes the accessory and structural proteins, including envelope (E), membrane (M), nucleocapsid (N), and spike (S) glycoprotein [3-5].
S protein is considered the essential structural protein of the COVID-19 virus. It mediates the viral binding to the human ACE2 receptor to facilitate the viral invasion into host cells. Inside the host cell, the S protein is cleaved by a host cell protease into S1 and S2 subunits. The S1 subunit mediates the interaction with hACE2, while the S2 subunit mediates the membrane fusion process [6, 7]. Binding of the Receptor Binding Domain (RBD) of the S1 subunit to hACE-2 receptor mediates the interaction of the heptad repeat 1 (HR1) and 2 (HR2) domains in its S2 subunit to make a six-helix bundle (6-HB) fusion core, bringing cellular and viral membranes in contact to facilitate infection [8]. The binding affinity of SARS-CoV-2 to ACE2 receptor is 10 to 20 times higher than SARS-CoV, which may be due to the presence of a distinct motif within the S1/S2 junction region responsible for viral fusion [7, 9]. Genome sequencing results and evolutionary analysis show that SARS-CoV-2 has 96.2% genomic similarity to Bat CoV RaTG1 virus. Therefore, bats are suspected to be the potential origin of the virus, which was transmitted later to humans by an unknown intermediate host [2], probably pangolins [10, 11].
1.2. Disease Transmission, Diagnosis, and Mortality Risk
The known route of transmission of the disease is respiratory droplets of patients while coughing or sneezing. The virus can also spread by contact with surfaces contaminated with the virus [12]. Virus transmission through feces was also recently reported [13]. The SARS-CoV-2 incubation period varies between 1 to 14 days [12], and the mean incubation period is 5.2 days [14].
The clinical characteristics of COVID-19 patients include fever which is the main symptom, dry cough, shortness of breath, fatigue, dyspnea, sore throat, and gastrointestinal symptoms such as vomiting, diarrhea, and nausea [15-17]. GI symptoms are not very common [16]. In most cases, the disease is mild with flu-like symptoms (80.9%). Severe cases requiring ICU administration represent 13.8%, while asymptomatic cases represent only about 1.2%, and they are usually children under 10 years [18]. Disease outcomes include respiratory failure, which is the major reason for death among patients (53%), circulatory failure, shock, fulminant myocarditis, acute respiratory distress syndrome (ARDS), renal complications such as AKI (75.4%), proteinuria, hematuria, multiple organ dysfunction, and death [16, 17, 19-21]. COVID-19 diagnosis is based on the detection of SARS-CoV-2 nucleic acids by RT-PCR in nasopharyngeal specimens [21]. Other critical laboratory measures for disease progression indication include white blood cells count, C-reactive protein (CRP), D-dimers, procalcitonin (PCT), LDH, and lactate levels [22]. Chest CT and X-ray Imaging on COVID-19 patients is also essential for disease diagnosis and prediction of its progression. Images show bilateral or unilateral pneumonia [23] and peripheral interstitial changes with different small patches of shadows in the early stages [21, 24]. In severe cases, chest images can show bilateral multiple ground-glass opacity, infiltrating shadows, and pulmonary consolidation, accompanied by infrequent pleural effusion [15, 21, 25].
To date, the reported susceptibility factors and fatal disease outcomes predictors include age [26, 27], chronic diseases such as diabetes [25, 28], cardiovascular diseases [18], hypertension [25, 29], cancer [30], chronic respiratory disease [18], and lifestyle habits such as obesity and smoking [31]. Viral load and viral-shedding period are also important predictors for disease severity and fetal outcomes among different age groups [32].
2. USING OLD DRUGS TO TREAT NEW DISEASES
Various pharmacological treatments have been employed for repurposing and are set to an evaluation in clinical trials to treat COVID-19, targeting various proteins in different phases of the viral life cycle. Some were already evaluated in randomized controlled trials (RCTs), while others still need adequate testing. In fact, repurposing aids in rapidly discovering treatments in a pandemic spread owing to their well-established safety profile [33]. Some of these drugs were excluded due to observed ineffectiveness, safety concerns in COVID-19 patients, or both, whereas some others showed auspicious results. Table 1 summarizes the repurposed drugs for COVID-19 treatment and their target proteins. The fact that 79.5% of the proteins encoded in the SARS-CoV-2 are genetically similar to those of other SAR-CoVs makes the previous drugs used for their treatment, in addition to broad-spectrum antivirals, possible for repurposing [34]. The pharmacological agents belong to (1) Antiviral drug classes like (a) Nucleoside analogues that target the RNA dependent RNA polymerase (RdRp), (b) HIV protease inhibitors, (c) Neuraminidase inhibitors; (2) Corticosteroids; (3) Biologic drugs like Tocilizumab and Sarilumab; (4) Antibiotic and antifungal agents that should only be used in cases like high procalcitonin, hospital-acquired pneumonia, ventilator acquired pneumonia and pneumocystis pneumonia; (5) Anticoagulants; (6) Fresh frozen plasma; (7) Other potential treatments including drugs blocking the renin-angiotensin system (RAS) are still under investigation [35].
Repurposed Drug | Target Protein in SARS-CoV-2 | PDB Code of the Crystal Structure and Resolution | Original Clinical Purpose | Discovery | Software Used | References |
---|---|---|---|---|---|---|
Protease Inhibitors | ||||||
Lopinavir | Main protease | 6Y2F Resolution: 1.95 Ao |
HIV | Molecular docking | IDentif.AI, DL (MT-DTI), ML (NLP, and NB), and AutoDock Vina | [49] |
Ritonavir | Main Protease | 6Y2F Resolution: 1.95 Ao |
HIV | Molecular docking | IDentif.AI, DL (MT-DTI), ML (NLP, and NB), and AutoDock Vina | [49] |
TMC-310911 | TMPRRS2/ 3C-Like proteinase | 6LU7 Resolution: 2.16 Ao |
HIV-1 | Docking-based virtual screening, molecular docking, and dynamics | PyRx 0.8 | [49] |
Camostat Mesylate | TMPRRS2 | 7MEQ Resolution: 1.95 Ao |
Serine protease inhibitor | Molecular Dynamics simulation and Markov modeling | PyEMMA 2 | [48] |
Nafamostat | TMPRRS2 | 7MEQ Resolution: 1.95 Ao |
Serine protease inhibitor | Molecular Dynamics | PyEMMA 2 | [48] |
Faldaprevir | 3C-Like proteinase | 6LU7 Resolution: 2.16 Ao |
HCV | Docking-based virtual screening, molecular docking, and dynamics | Surflex-dock and Autodock Vina | [43] |
Aclarubicin | 3C-Like proteinase | 6LU7 Resolution: 2.16 Ao |
Cancer drug with multiple mechanisms of action | Docking-based virtual screening, molecular docking, and dynamics | Surflex-dock and Autodock Vina | [38] |
Saquinavir | 3C-Like proteinase | 6LU7 Resolution: 2.16 Ao |
HIV | Docking-based virtual screening, molecular docking, and dynamics | Surflex-dock and Autodock Vina | [38] |
Neuramidase Inhibitors | ||||||
Zanamivir | 3C-Like proteinase | 6LU7 Resolution: 2.16 Ao |
Influenza A & B | Molecular docking-based virtual screening & Homology modeling | Schrodinger® | [52] |
Peramivir | 3C-like proteinase | 6LU7 Resolution: 2.16 Ao |
Influenza | Molecular docking and Molecular dynamics | Molecular Operating Environment (MOE) & GROMACS | [52] |
Oseltamivir | 3C-like proteinase | 6LU7 Resolution: 2.16 Ao |
Influenza A & B | Molecular docking and Molecular dynamics | Molecular Operating Environment (MOE) & GROMACS | [53] |
Nucleoside Analogue | ||||||
Favipiravir | RdRP | 6L4R Resolution: 2.147 Ao |
Influenza | Molecular docking & structural modeling | AutoDock Vina & MODELLER 9.23 | [36] |
Galidesivir | RdRP | 6L4R Resolution: 2.147 Ao |
HCV | Molecular docking | Autodock Vina | [38] |
N4-Hydroxycytidine | 3C-like protease | 6LU7 Resolution: 2.16 Ao |
Broad-spectrum antiviral | Molecular docking | Autodock Vina, GOLD | [40] |
Remdesivir | 3C-like protease | 6LU7 Resolution: 2.16 Ao |
Broad-spectrum antiviral | Molecular docking | IDentif.AI, DL (MT-DTI), ML (NLP), and AutoDock Vina | [36] |
Ribavirin | RdRP | 6L4R Resolution: 2.147 Ao |
RSV and HCV | Molecular docking | Autodock Vina | [37] |
Triazavirin | Main Protease | 6Y2F Resolution: 1.95 Ao |
Broad-spectrum antiviral | Molecular docking and molecular dynamics | Autodock Vina & MMGBSA approach | [39] |
EIDD-2801 | RdRP | 6L4R Resolution: 2.147 Ao |
Influenza Virus | Docking-based virtual screening, molecular docking and dynamics | Guassian 16 | [40] |
2.1. Repurposing of Antiviral Drugs
2.1.1. Nucleoside Analogues
The nucleoside analogues repurposed for COVID-19 are analogues for either guanine, adenosine, or cytidine, and they all target RdRp aiming to block the viral RNA synthesis. The drugs tested in RCTs and non-RCTs are Remdesivir, Favipiravir, Galidesivir, Ribavirin, Triazavirin, GS-44124, N4-Hydroxycytidine, and its prodrug EIDD-2801 [36-42]. The tested drugs in RCTs are Remdesivir, Favipiravir, and Galidesivir; though Galidesivir RCT is currently recruiting patients (NCT03891420) with no preliminary results available yet [43]. In fact, the most promising drug for the treatment of COVID-19 is Remdesivir. It showed a significant decrease in the viral load despite some safety concerns. In the last preliminary report of the RCT conducted to investigate Remdesivir (NCT04280705), a shorter recovery time of 11 days was observed in patients requiring oxygen who received Remdesivir as compared to the group of patients that received the placebo (15 days recovery time). Adverse events were observed in 21.1% of patients who were randomized to Remdesivir, compared to the placebo group of 27% adverse events [44]. Currently, the Food and Drug Administration (FDA) has issued an emergency use authorization (EUA) for Remdesivir for the treatment of adult and children COVID-19 patients [45]. From a pharmacological scope, Remdesivir is a prodrug that is metabolized into an alanine metabolite upon entry to the cell (GS-704277) that is afterward processed into a monophosphate derivative and then to the active nucleoside triphosphate derivative. It acts as a competitive inhibitor for the incorporation of native adenosine triphosphate into the growing polynucleotide chain. Moreover, it displays linear pharmacokinetics with a long half-life (>35 for the parent drug) [46]. Another RCT for Favipiravir (ChiCTR2000030254) showed that patients who received the drug had shorter latencies for fever and cough relief than patients who took another repurposed drug (Umifenovir), which will be discussed later in this review. Also, it demonstrated a clinical recovery rate of 7 days. The only adverse effects observed were a rise in serum uric acid level that is mild and manageable [47]. Another RCT is currently conducted to assess Favipiravir in combination with Nafamostat Mesylate (an anticoagulant discussed later in this review) and to compare this combination with administering Favipiravir alone [48] (Fig. 1).
2.1.2. Protease inhibitors
Some protease inhibitors were assigned for repurposing to treat COVID-19 owing to their potential ability to prevent the maturation of major proteins in the viral life cycle. These drugs are lopinavir/ritonavir combination, Darunavir, TMC-21091, and TMC-210911 [49]. In silico studies of drug-enzyme binding have shown potential interactions between 20 protease inhibitors and the main SARS-CoV-2 protease. Accordingly, Other HIV protease inhibitors have been selected as drug candidates using computational methods but did not yet undergo in vitro tests or clinical trials.
Even though the lopinavir/ritonavir combination showed an effective inhibition in vitro, an RCT (ChiCTR2000029308) aimed to investigate its effect on the late stage of COVID-19 patients with oxygen saturation (SaO2) of 94% or lower, compared to patients receiving standard care alone, showed no benefit beyond the standard care. Since this RCT was performed on patients with a late stage of pulmonary infection, it might be misguided to conclude that lopinavir/ritonavir has no benefit whatsoever because other severity stages like the first stage of viral replication or immune hyper-reactivity were not assessed against this drug combination [50, 51] (Fig. 2).
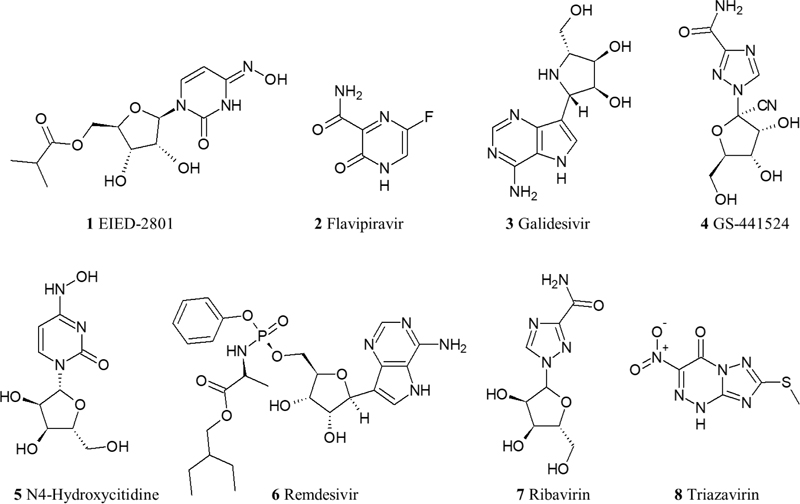
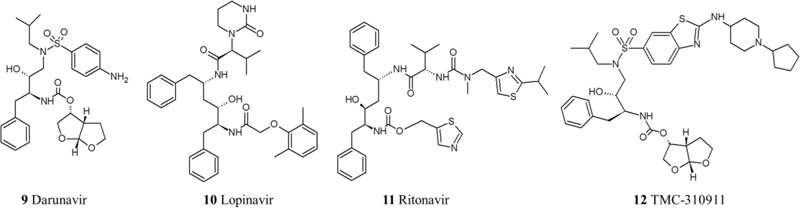
2.1.3. Neuraminidase Inhibitors
Neuraminidase is an essential enzyme in the influenza viral life cycle. A sequence comparison study found a similarity in the neuraminidases of H1N1, NS1, and NS2 of SARS-CoV-2. Based on this study, neuraminidase inhibitors have been suggested for repurposing to treat COVID-19. However, there are some concerns about the viral resistance for these agents. To date, there is no sufficient evidence for the effectiveness of neuraminidase inhibitors for the treatment of COVID-19. But, CDC has recommended the repurposing of three neuraminidase inhibitors; Oseltamivir, Zanamivir, and intravenous Peramivir [52, 53] (Fig. 3).
2.2. Repurposing of Corticosteroids
Corticosteroids are thought to be among the most important adjuvant therapies for reducing the severity and mortality of COVID-19. The late stage of the disease leads to cytokine storms that consequently cause damage to multiple organs. It has been reported that dexamethasone and methylprednisolone have positive impacts on improving the dysregulated immune responses and regulation of hypotension. In a retrospective cohort study, 201 COVID-19 patients who developed ARDs and were treated with methylprednisolone showed a significant reduction in the mortality rate [54]. Another study showed a strong correlation between the administration of methylprednisolone and both improved symptoms and reduction in latency in patients who developed acute respiratory failure and showed symptoms like fever and hypoxia, compared to patients who did not receive the drug [55]. In addition, Dexamethasone has shown a reduction in mortality and the ventilation period in ARD patients. More evidence and larger-scale studies are still needed (Fig. 4).
2.3. Antibiotic and Antifungal Agents
Antibiotic agents should only be used in the case of procalcitonin (PCT); as a result of ventilator-associated pneumonia or hospital-acquired pneumonia. Non-RCTs trials showed the effectiveness of Teicoplanin in the treatment of COVID-19 as it potently blocks the entry to the cell [56]. In addition, Azithromycin has been tested but showed cardiac toxicities in the form of prolongation of QT interval [57]. Also, an observational study has shown that doxycycline improves respiratory symptoms and COVID-19 associated anosmia [58] (Fig. 5).
2.4. Anticoagulants
It has been found that there is a variation between reported incidences of Venous Thromboembolism (VTE) in hospitalized COVID-19 patients. VTE therapy should be given to adult hospitalized patients only per the standard care for hospitalized adults.
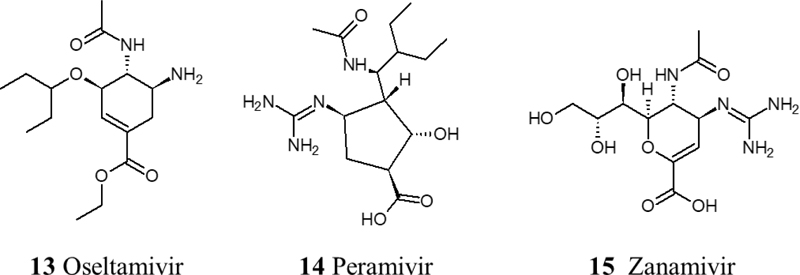
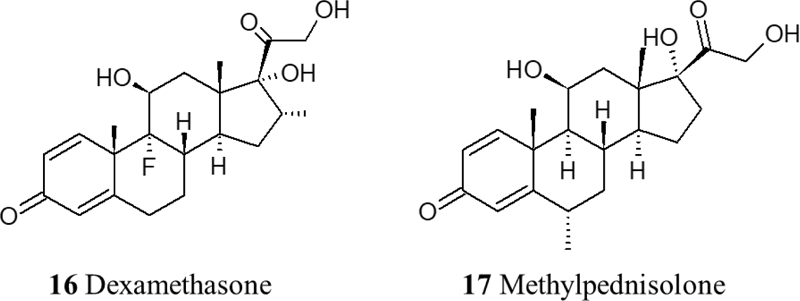
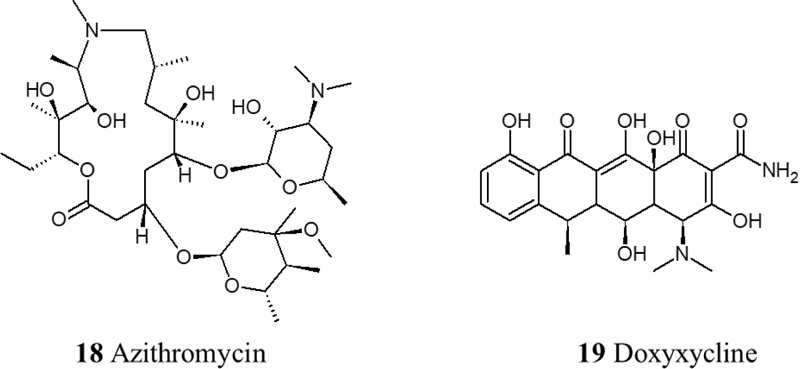
Data from various clinical trials associate infection of SARS-CoV-2 with a prothrombotic state, with increases in fibrin, fibrinogen, fibrin degradation products, and D-dimers [59, 60]. Therefore, anticoagulant therapy is crucial in treating COVID-19 due to the hypercoagulable state that has been observed in COVID-19 patients that consequently leads to different stages of thrombosis. Low molecular weight heparin therapy has shown a reduction in mortality by prevention or treatment of thrombosis in severe COVID-19 patients [61]. Moreover, Heparin can contribute to the inhibition of viral attachment owing to its potential in causing conformational changes to spike S1 of SARS-CoV-2, in addition to Heparin’s anti-inflammatory properties [62]. Another therapeutic approach to inhibit the thrombo-inflammatory response is the inhibition of the contact factor inhibitory pathway targeting coagulation factor XII. Using an FXIIa inhibitory antibody is a potential therapy in this approach. In fact, it is currently being investigated in a clinical trial (NCT03712228). Also, fibrinolysis therapy has shown promising results using plasminogen activator (tPA), a fibrinolytic agent that converts inactive plasminogen into plasmin which in turn breaks down cross-linked fibrin. In a recent clinical trial, it has been observed that nebulized fibrinolytic therapy correlates with better oxygenation and ventilatory parameters [63]. Recently, agents with antiplatelet effects like dipyridamole and Nafamostat have shown effective results in in vitro studies by suppressing SARS-CoV-2 and previously MERS, respectively [64, 65]. Nafamostat is a serine protease inhibitor with antiplatelet effects and is known to inhibit TMPRSS2 [66]. Currently, the RACONA RCT (NCT04352400) is aiming to investigate the efficacy of Nafamostat in severe COVID-19 patients (Fig. 6).
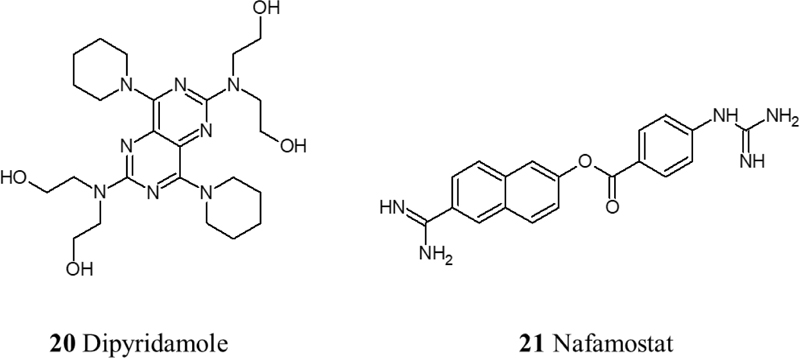
3. IMMUNE-BASED THERAPY
3.1. Convalescent Plasma and Immunoglobulins
Convalescent Plasma (CP) is the plasma donated from already cured patients that include antibodies against SAR-CoV-2. In addition, concentrated immunoglobulin preparations from the plasma of recovered patients have also been used. The CP therapy has been effective in SARS, MERS, and 2009 H1N1 pandemics [67-70]. The high virological and clinical data similarity between these viruses and SARS-CoV-2 strongly promoted trying CP therapy for the treatment of COVID-19. A pilot study was conducted on 10 severe COVID-19 patients with various symptoms. The results were that all symptoms of the 10 patients, including fever, cough, chest pain, shortness of breath, vastly improved from 1 to 3 days. Moreover, chest CT examinations showed a reduction in pulmonary lesions with much better pulmonary function. Also, 5 of the 9 patients achieved an increase in the amount of the neutralized antibody titers. Before the CP transfusion, 3 of the 10 patients were tested negative of the SARS-CoV-2 RNA by qRT-PCR assay, while the other 7 were tested negative after CP transfusion, three of them on day 2, the other three on day 3, and the last one on day 6. No serious adverse effects of CP therapy were observed. The results achieved show the effectiveness of using CP therapy for the treatment of COVID-19 [71].
3.2. Interleukin-1 Inhibitors
One of the signs of COVID-19 is the elevation of endogenous Interleukin-1 (IL-1) along with cytokine release syndrome. Thus, using IL-1 inhibitors is of potential therapeutic value [72]. A recent case report stated that subcutaneous Anakinra (an IL-1 receptor antagonist) treated COVID-19 as a monotherapy. It reports a rapid and continuous improvement of respiratory manifestations and fever. After 10 days, the inflammatory parameters of the patients were improved. On day 19, the patient was discharged with no need for oxygen supplementation, and the chest CT showed improvement of the pulmonary infiltrates [73].
3.3. Interleukin-6 Inhibitors
It has also been found that elevation of IL-6 is a normal response of severe systemic inflammatory response in severe COVID-19 patients [74, 75]. This makes the IL-6 blockade of therapeutic significance. The following IL-6 inhibitors were tested; Sarilumab, Siltuximab, and Tocilizumab. A clinical trial to investigate the effect of Sarilumab (NCT04315298) showed a significant reduction of the C-reactive protein levels and the overall mortality rate. Adverse effects included reversible elevations in liver enzymes, seldom occurrences of neutropenia, and thrombocytopenia. Some risk of serious infections was also reported in the case of long-term usage of Sarilumab. Regarding Tocilizumab, an RCT (NCT04331808) showed that it improved symptoms and had a great effect on reducing the mortality rate. However, detailed results have not been published yet [76].
3.4. Interferons
Interferons alfa/beta are only recommended for clinical studies and not for treatment purposes. An RCT using interferons in combination therapies on 301 patients showed no improved symptoms and had no benefit over the standard care [77]. Therefore, more research should be done to reach a conclusion on their effect.
4. BIOINFORMATICS
Once the data of the SARS-CoV-2 genome were published on The National Center for Biotechnology Information (NCBI) and Global Initiative on Sharing All Influenza Data (GISAID) database, various studies related to bioinformatics analysis had appeared providing significant information for the SARS-CoV-2 treatment. Bioinformatics analysis helps to determine the gene structure, evolution, mechanisms, and function, as well as prediction of possible epitopes. The combination of bioinformatics analysis, epidemiological data, and experimental outputs may give an indication for improving the selection of an animal model for in vivo experiments [78].
4.1. Network Bioinformatics Analysis
The gaps in the information of COVID-19 have to be filled up by the quick sharing of relevant data [79]. In the United Kingdom (UK), a network called “COVID-19 Genomics UK (COG-UK) Consortium” was launched to provide sequence data for 230 thousand SARS-CoV-2 samples from different patients [80]. In addition, various bioinformatics networks were established for the investigation of drug repurposing [81, 82]. Another approach is to build a network model for the viral-host interactome using protein-protein interactions for previous HCoV S-glycoprotein to study the pathogenicity of SARS-CoV-2 [83]. The master regulator analysis was conducted to predict most of the human interactome influenced by infection [84]. The network-based on gene interaction and pathway tracking has assisted in obtaining a reference for clinical studies [85]. A group from the University of Alabama developed a network including multi-omics data called “Calu-3-specific human-SARS-CoV-2 Interactome” which comprises information about novel molecular components and pathogenicity [86]. Another group adopted a systems biology approach that accesses precious information about miRNA data and transcriptome, yielding an understanding of the binding and replication machinery for SARS-CoV-2 [87]. The Network bioinformatics analysis has a significant role in finding a treatment for COVID-19 by predicting 24 pathways, 34 genes, 5 modules, and more than 30 drugs that could be potentially repurposed [88,89].
4.2. Evolution and Conservation
The replicative machinery is evolving in SARS-CoV-2 at a high rate, which can produce different mutations along the genome [90]. There was no evidence for intra-host mutation until the end of 2020 [91]. In 2021, this evidence appeared in patients worldwide and should not be neglected [92-94]. The genotyping analysis for SARS-CoV-2 was applied using fast multiple sequence alignment followed by directed Jaccard distance as an approach to provide information about the presence of mutations and Single Nucleotide Polymorphisms (SNPs) [95]. The mutation in essential proteins such as RdRp [96], Non-Structural Protein 6 that has a role in viral autophagy [97], and S protein [98] and the deletion [99] should be considered during the development of effective treatments. This is also important for designing a set of primers for the diagnosis of the SARS-CoV-2 using reverse-transcribed quantitative PCR (RT-qPCR) [100]. The conservation domains in other viruses such as poliovirus and bronchitis with SARS-CoV-2 polypeptide-1 can be significant clues for developing a vaccine [101]. On the other hand, the phylogenic tree is a powerful tool to estimate the origin of the virus and predict if the evolution occurred by nature or in the laboratory [102, 103]. Various classifications were made according to the occurrence of SNPs in the genome, such as the S and L lineages, by noticing the orf1ab T8517C, sense mutation, and ORF8 C251T [104]. By using the single nucleotide variations, Yang et al. classified the virus into six phylogenetic clusters with a relation between the mutation and the mortality rate [105]. Another study has used SNPs for classifying the virus into four groups. One of them was proved more aggressive [106]. The trials started to design a barcode of 10 SNPs in the genome to facilitate the tracking process [107]. In addition to SNPs, the recombination and insertions specifically at the cleavage site of the receptor-binding domain may increase the ability to infect the host cell [108].
4.3. Prediction of Epitopes
Identification of the part of the virus genome that is recognized by immune responses in the human gives a great opportunity for designing a new vaccine and assists in evaluating vaccine candidate immunogenicity. To date, various studies are being conducted to predict T and B cells epitopes using different bioinformatics tools. The strategy of finding conserved parts in the viral genome with newly acquired adaptations can be used to generate hypothetical B cell epitopes [109]. The Immune Epitope Database and Analysis Resource (IEDB) [110] and The Virus Pathogen Resource (ViPR) [111] are two resources for known epitopes. They were used to predict candidate targets for SARS-CoV-2 based on previously known epitopes from other coronaviruses [112]. In addition, the BepiPred 2.0 server has recently been used to identify B-cells epitopes for the virus [113, 114]. The strategy based on 9-mer epitope prediction can provide epitopes through HLA class I alleles [115]. The conservation between SARS-CoV and SARS-CoV-2 in S and N proteins was a significant starting point to produce preliminary data for T cells epitopes [116]. The X-ray crystal structure of the conserved cryptic epitope (CR3022), which is known as a neutralizing antibody for SARS-CoV [117], in complex with the S protein of the SARS-CoV-2 may highlight its role as an essential target [118]. However, around 24.5% of amino acids in S protein are different from SARS-CoV which opens the track to predict unique antibody epitopes that may have a significant effect [119].
5. MOLECULAR MODELLING
In the absence of effective treatments or vaccines against COVID-19, and because of the adverse impact the COVID-19 brought on the work environment worldwide, the role of computational approach and in silico studies dramatically emerged. Structural biology, homology modeling, and molecular docking exhibited a fundamental role in the ongoing trials for the discovery of COVID-19 therapy.
5.1. Structural Biology
Elucidation of the 3D structure of SARS-CoV-2 essential subunits has a substantial role in drug discovery, particularly through molecular docking. Therefore, many scientists dedicated their time and effort to resolve the crystal structure of vital proteins in SARS-CoV-2 as well as the binding pockets and the key amino acids. This would pave the way for structure-based drug design for COVID-19 treatment. The determination of the 3D crystal structures of SARS-CoV-2 proteins was performed mainly by X-ray crystallography (XRD) [34, 118, 120-130]. Also, Cryogenic Electron Microscopy (Cryo-EM) produced some 3D structures but less than those obtained from XRD [131-139]. On the other hand, Nuclear magnetic resonance (NMR) had a minor role in detecting SARS-CoV-2 crystal structures. Till October 2020, there was only one protein structure of this virus obtained using NMR, representing the nucleocapsid protein (N) (PDB ID: 6YI3). However, during the first half of 2021, the number of structures was increased by 3, which can be found in the protein data bank as solved structures using solution NMR under the Source Organism Taxonomy Name = “SARS-CoV-2” in the search bar. The 3D structures have been elucidated for many protein types in SARS-CoV-2, either structural or Nsps Table (S2). One of the most important structural proteins detected in the 3D form is the S protein obtained by Cryo-EM [132-134]. Additionally, another study elucidated the S protein by Cryo-EM in a closed state (PDB: 6VXX) and an open state (PDB: 6VYB) [135]. Another resolved structural protein of SARS-CoV-2 is the N protein, obtained using XRD (6WZO, 6WZQ, and 6M3M) and NMR (PDB: 6YI3) [121, 122]. On the other hand, the Nsps detected include RdRp, which was obtained by Cryo-EM [137, 138].
Since the main protease (Mpro), also known as 3C-like Main Protease (3CLpro), is a versatile drug target, many crystal structures were prepared and submitted to the PDB, and many of them are still from unpublished data [123-126]. The most commonly used Mpro crystal in the process of structure-based design is 6LU7 [123]. Several crystal structures were also published for papain-like protease (PLpro) and NSP15 [127, 128]. It is worth noting that some viral protein structures were determined as a portion of a complex, such as NSP12-NSP7-NSP8 obtained by Cryo-EM [139]. The elucidated proteins are crystallized, in varying qualities, either alone in an unbound state or complex with a ligand (Table S1, updated until 31 of March 2021 Supplementary Data). The role of structural biology can be significant in predicting the architecture of the SARS-CoV-2 proteome [140].
The Protein data bank returns 1232 structures after using Source Organism Taxonomy Name = “SARS-CoV-2” as a keyword for search, updated 26 May 2021. The data was distributed among X-ray diffraction, Electron microscopy, Solution NMR, Solid-state NMR, and Neutron diffraction, and the numbers of structures were 898, 330, 3, 2, and 1, respectively. It was obvious that X-ray is the most abundant tool worldwide due to the high resolution of the structures with refinement resolution <2 Å that is only found among x-ray structures. Those data come up with this unusual speed as a result of extensive partnership and collaboration around the world in a search to find a solution to stop the SARS-CoV-2 breakout [79]. However, unfortunately, the more quickly work is done, the more errors and incorrect information appear. Several structures were studied and submitted in non-peer-reviewed journals that build a large library of misleading information, and this issue was discussed in detail with requests to enhance SARS-CoV-2 structures elsewhere [141].
The computational works in structural biology can be extended to include predicting the 3D structures by using various tools, including AlphaFold as an Ab initio tool based on deep neural networks [142] that have been used in predicting ORF8 [143], NSP6 [144], or other SARS-CoV-2 proteins [145]. Although this technique is promising in the case of building novel proteins, the high computational cost makes homology modeling crucial.
5.2. Homology Modeling
Due to the rapid mutation in the SARS-CoV-2 and since the process of crystallization consumes time and effort and is not feasible in certain cases, many scientists exploited computational approaches to predict the structure of specific proteins in SARS-CoV-2 through homology modeling. This is done by first retrieving the sequence of the virus target protein and aligning this sequence with the experimentally solved proteins with the best homology. Since there is high conservation between SARS-CoV-2 and SARS-CoV, around 80% nucleotide sequence identity [146], the SARS-CoV 3D proteins are usually used as templates to perform the protein modeling. Once the sequence alignment is available, homology modeling software uses a specific algorithm to fold the target protein using structural information obtained from the template.
A recent study attempted to predict the 3D structure of SARS-CoV-2 E protein from its sequence in NCBI, accession number YP_009724392, using the ITASSER server and exploiting the corresponding protein from SARS-CoV (PDB ID 5X29) as a template [147]. This protein was used as a template due to the conservation with the SARS-CoV-2 E protein with 88.71% sequence identity [147]. Regarding the M protein of SARS-CoV-2, a study generated its 3D model, based on SARS-CoV M protein, using its sequence from NCBI, Accession number QHD43419, and the ITASSER server [147]. Similarly, the S protein 3D model was developed using SAR-CoV S protein as a template and Modeller software [148-150]. Also, the 3D model of the RdRp protein structure has been built using the SWISS-MODEL server and the corresponding SARS-CoV protein as a template [149, 151, 152]. The 3D models for some SARS-CoV-2 proteases such as PLpro and Mpro were also developed using the SWISS-MODEL server and Modeller software [149, 152]. Finally, the 3D structure of Guanine-N7 methyltransferase, known as nonstructural protein 14 (nsp14), was predicted by using Modeller software based on SARS-CoV nsp14-nsp10 complex (PDB ID 5C8S) [153].
The spike and Orf8 for the new variant SARS-CoV-2/B.1.1.7 were predicted by the Swiss model for antibody binding site prediction [154]. In this study, the template was solved using Cryo-EM that has a lower resolution compared to other experimental tools [155]. ACE2 protein sequence homology was used to estimate the risk of SARS‐CoV‐2 infection in animals. However, the authors did not support this hypothesis with reported cases [156]. Overall, the results coming from predictions based on another prediction are not preferred in science as the percentage of error becomes high.
5.3. Molecular Docking and Virtual Screening
The process of drug discovery consumes significant time and resources until it reaches the clinical trial stages before introducing approved drugs to the market. Therefore, molecular docking has been employed to suggest potential lead compounds that are likely to target SARS-CoV-2 specific proteins or host cell proteins essential for viral entry and survival. These candidates are not guaranteed to have an actual efficacy toward the targets until in vitro and in vivo validations are available.
Several studies performed docking using FDA-approved drugs with known efficacy and toxicity [149, 151, 157-160]. Other studies investigated the interaction of natural products or extracts with various SARS-CoV-2 proteins [147, 161-166]. Moreover, scientists exploited several libraries of commercially available drug-like compounds for docking into the viral proteins. The most prominent databases used are PubChem [162, 163], ZINC database [149, 152, 160], ChemSpider [157, 165], Drug Bank database [158], TIPdb database [166], Indian Medicinal Plants, and Phytochemistry and Therapeutics (IMPPAT) database [147]. The most commonly used docking programs to detect the binding affinity between the protein target and the compounds are AutoDock Vina [147, 151, 152, 161, 165], GOLD suite [161], Schrodinger glide docking module [148, 159, 162, 167], Swiss Dock [157], AutoDock [158, 166], and idock [164]. The aforementioned programs utilize algorithms to sample the conformational space of the protein-ligand complexes to identify the accurate binding mode, followed by calculating the affinity score of the complex to determine the low energy configurations as the accurate binding mode [168, 169]. However, they act differently about the algorithms used, the scoring function, and the protein-ligand representation [168, 170].
The target structure is either retrieved from Protein Data Bank (PDB) or predicted by homology modeling. There are a large number of molecular docking studies targeting SARS-CoV-2 structural proteins such as S protein [148, 149, 162, 165], envelope protein (E) [147, 166], membrane protein (M) [147], and N protein specifically the N-terminal domain (NTD) [167]. As mentioned above, spike (S) protein is the main tool used by the virus for entry into the host cell. Therefore, several studies aimed at predicting the interaction of this protein with host receptors as well as with small candidate ligands [162, 162]. The databases used for docking include the well-known ZINC database of commercially available compounds, a library of natural products, and in-house libraries of the developing companies. Additionally, Nsps such as PLpro or Nsp3 [149, 158], Mpro, and Nsp5 were also used for virtual screening [148, 149, 152, 157, 159-161, 163]. The viral main protease (Mpro) is a cysteine protease responsible for cleaving the large polyprotein at more than ten sites, usually at the amino acid sequence Leu-Gln-(Ser, Ala, Gly). This protein acts as a dimer with a limited catalytic activity of the monomers. To date, no Mpro inhibitors have been approved by the FDA for treatment of COVID-19, and only Pfizer reported a ligand, PF-07304814, with activity on this enzyme. There are a large number of reported crystal structures of this viral protein and a surprising number of in silico studies describing the discovery of inhibitors for the treatment of COVID-19 [124-127. 157-163]. Most of the recent studies focus on the screening of FDA-approved compounds. However, we noticed that in most cases and despite the conclusive titles, these studies are merely theoretical and are not validated by biological evaluation. We believe that, under this pandemic situation and with the increasingly available and more affordable assay formats, all these virtual screening campaigns should be concluded with an in vitro protease assay.
Notably, Papain-like protease (PLpro) is also considered an important drug target because of its substantial role in polyproteins cleavage. The PLpro catalyzes the proteolytic cleavage at nsp1/2, nsp2/3, and nsp3/4. It is a cysteine protease that has the conserved catalytic triad Cys111-His272-Asp286, which targets the P1−P4 sequence GGXL [127]. The PLpro is the least explored target among the characterized molecular targets of the SARS-CoV-2, relative to the 3CLpro on which extensive studies have been made, the RdRp, which is the target of the only FDA-approved drug for Covid-19 treatment, and the S protein used in vaccine development. In a previous “all-theoretical” study, the authors suggested repurposing some FDA-approved drugs as PLpro inhibitors, however, without offering any biological evaluation or even molecular dynamics (MD) explanation. Other studies proposed novel potential inhibitors of the PLpro, but the lack of cellular antiviral confirmation is considered a substantial limiting step in most of these studies [171].
Furthermore, molecular docking was also performed into viral Nsp15 endoribonuclease (NendoU) [162], RdRp, Nsp12 [149, 151, 152, 164], and helicase (Nsp13) [149, 152]. On the other hand, a host cell protein structure could be a drug target to inhibit the SARS-CoV-2 infection. These host cell targets include Angiotensin-Converting Enzyme 2 (ACE2) receptor, which binds to the viral S protein during cell entry [149, 165], and TMPRSS2, which is a protease essential in S protein cleavage and facilitates viral entry [149].
Currently, molecular docking studies are validated by molecular dynamics simulations for further understanding of the binding interactions. It is nearly the gold standard procedure in SARS-CoV-2 molecular dynamics drug repurposing research. For example, Muralidharan and colleagues used AutoDock to search for potential inhibitors for MPro. In addition, they simulated each one of the obtained three proteins and all of them together. The molecular dynamics simulations revealed the raising binding potential of lopinavir, oseltamivir, and ritonavir with the MPro from –5.11 to –8.32 kcal/mol [172]. Generally speaking, molecular docking methods are a broadly active area of research, particularly with the lack of resources and time in the era of the pandemic (Fig. 7). For example, high throughput screening to obtain the best hits was done based on 1.3 billion compounds that were docked against MPro using a novel technique called “Deep docking” [173]. Also, the interaction between nanoparticles and different proteins was identified using molecular docking [174]. However, there is insufficient data regarding using the docking tool to predict the interaction between nanoparticles and SARS-Cov-2, especially after confirming the antiviral role of nanoparticles [175, 176]. The process of docking was extended to include the peptide-protein interaction between nisin, a peptide that works as an antimicrobial in food preservation, and human ACE2 [177, 178]. This work opens the track to use our food as a significant tool in curing the disease. A recent study predicted the protein-protein interaction between neural proteins such as Aβ, α-synuclein, tau, prion, and TDP-43 RRM and SARS-CoV-2 Spike S1 protein receptor using docking [179]. This work illustrates the importance of docking in explaining the reasons behind the high risk of catching a neurodegenerative disease for people infected with SARS-CoV-2 [180]. Due to the significant role of docking studies, several databases specific for SARS-CoV-2 docking results should be built and made available to the public in order to accelerate the COVID-19 emergency drug discovery. For example, various ligand-protein and protein-protein interactions could be easily accessed throughout the user-friendly free-to-access SARS-CoV-2 3D database at https://sars3d.com/ [181].
5.4. Pharmacophores and QSAR Models
Ligand-based drug design approaches depend on using information gained from molecules active on the target of interest to provide guidance for the design of novel ligands. Typically, several ligands with high affinity to the protein target are required, while the 3D structure of the target is not necessarily solved. Since the outbreak of COVID-19, many research groups have developed both ligand- and structure-based pharmacophores as well as QSAR models to describe the binding determinants of the biological targets that could be targeted for the treatment of this disease. We will provide examples of the models developed for each biological target. However, this is not meant to be an exhaustive list. As mentioned above, the SARS-CoV-2 main protease (Mpro) draws great attention with several pharmacophore models reported. Recently, Saeed et al. have developed a receptor-based pharmacophore model for the MPro for identifying FDA-approved drugs and hits from natural products [182]. This model was based on the MPro crystal structure (PDB ID: 6LU7) and consisted of a hydrogen bond acceptor, donor, and hydrophobic features. Similarly, scientists from UC San Diego have reported a structure-based pharmacophore of the SARS-CoV-2 RDRp based on its crystal structure with Remdesivir (PDB ID: 7BV2) [182]. This “huge” model consists of two acceptors, three donors, one acceptor and donor, three donors or acceptor, one hydrophobic, and an excluded volume of R=1.1 Å. In another study published in May 2021, a Korean team developed a structure-based pharmacophore for the S-adenosyl methionine binding site of the nsp10-nsp16 complex based on its crystal structure (PDB ID: 6W4H) [183]. Their best model was composed of 2 hydrogen bond acceptors, 2 hydrogen bond donors, and 2 positive ionizable to screen the DrugBank database resulting in three hits. On the other hand, a ligand-based pharmacophore was also developed for the host protein Transmembrane serine protease 2 (TMPRSS2), based on four serine protease inhibitors (camostat mesylate, nafamostat, pefabloc SC, and phenylmethylsulfonyl fluoride) and two Janus-associated kinase inhibitors (baricitinib and ruxolitinib). The selection of these compounds was based on previous reports of promising anti-COVID-19 activity. However, the authors did not provide convincing evidence of the relationship of these agents to the TMPRSS2. This model was then used for screening the ZINC database for commercially available hits [184].
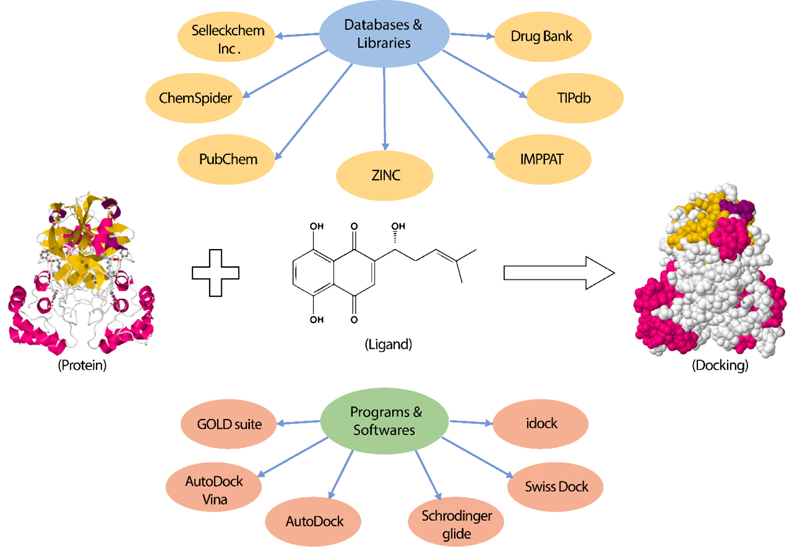
It is worth noting that the majority of these studies prioritized certain hits based on virtual screening, docking, and/or molecular dynamics simulation without performing in vitro biological evaluation against the studied protein targets. We perceive this as a serious limitation that undermines the results of these studies, and we suggest that future studies should be complemented by enzyme assays. These assays are getting more affordable over time due to the increasing interest of the scientific community in studying SARS-CoV-2 biology. Furthermore, most of the reported pharmacophores, especially those of viral proteins, are based on crystal structures (structure-based) rather than a collection of active ligands. Structure-based pharmacophores usually possess too many chemical features making them too restrictive and sub-optimal for virtual screening purposes. Moreover, these models cannot reflect the quantitative structure-activity relationship (QSAR) and, hence, cannot be used for activity prediction because they are derived from a single macromolecule–ligand complex.
Similarly, several QSAR models were developed to describe the electronic and steric features of the ligands of various proteins targeted for COVID-19 treatment. For example, Tejera and co-workers used a machine learning strategy to generate a QSAR model based on hundreds of inhibitor molecules of the main protease (Mpro) of the SARS-CoV coronavirus (the virus responsible for the 2003 outbreak) [185]. These compounds were selected due to the limited number of ligands reported for the new SARS-CoV-2 and, also, the significant similarity between the structure and sequence of SARS-CoV and SARS-CoV-2 Mpro proteases. The authors used the CHEMBL database to retrieve all the compounds reported to interact with the SARS-CoV Mpro. Then, they used a subset of all the ligands with an IC50 of less than 10 µM. This model was subsequently used for screening DrugBank, and the hits were then docked into the SARS-CoV-2 MPro. Finally, the authors suggested twenty hits for further study. In another interesting study, scientists from the University of North Carolina developed QSAR models based on 113 compounds with known activities against SARS-CoV MPro. Similarly, the research team collected all the ligands with reported activity on the SARS-CoV MPro in the CHEMBL database and used a threshold of 10 µM to differentiate them into actives (40) and inactives (73). The authors employed several QSAR techniques, including SiRMS, which uses fragment-based descriptors including several physical-chemical parameters, such as atoms’ lipophilicity and partial charges, and Dragon, which adopts whole-molecule descriptors; constitutional, topological, and geometric. These models were used to screen 9,615 compounds in the DrugBank database and yielded 42 Hits. Subsequently, the National Center for Advancing Translational Sciences (NCATS) tested 11 of these 42 hits, and three of them, cenicriviroc, proglumetacin, and sufugolix showed micromolar activities against the SARS-CoV-2 MPro [186]. There is no doubt that the MPro is an important and promising target for fighting COVID-19. We believe that depending on the SARS-CoV MPro ligands for the design of inhibitors of the SARS-CoV-2 enzyme was justified during the early days of drug discovery for this pandemic. However, with the increasing number of agents acting on the SARS-CoV-2 main protease, we think that future QSAR studies should depend merely on SARS-CoV-2 ligands. In addition to the MPro QSAR models, Amin et al. used 91 known inhibitors of the SARS-CoV PLPro to create a total of 21 models based on SMILES and graph-based descriptors. The best model was then used to screen an in-house library of small molecules and the top hits were docked into the crystal structure of the SARS-CoV-2 PLPro. This approach resulted in prioritizing thirteen compounds for further biological evaluation [187]. Interestingly, scientists from Lanzhou University, China, developed a QSAR model for 27 natural products based on their non-specific antiviral activity against SARS-CoV-2 without information about binding to certain biological targets. This model was based on two descriptors; Final Heat of Formation/number of Atoms (FHFA) and Max Antibonding Contribution of a Molecular Orbital (MACMO), and showed a correlation coefficient of 0.91 [188].
Despite the significant number of the QSAR models describing the activity of a large number of compounds towards various SARS-C0V-2 targets, none of these models was actually used for actual drug discovery campaigns complemented by biological evaluation. Again, these studies aimed at recommending certain hits for further future studies. In addition, due to the relatively low number of reported compounds with in vitro activities towards SARS-CoV-2, a lot of these QSAR models were based on activity data on the SARS-CoV of 2003 outbreak and tried to extrapolate their conclusions to the SARS-CoV-2. This also represents a serious limitation, and we recommend building future QSAR models using biological data from SARS-CoV-2 assays only because these data became more available and usually freely accessible.
5.5. The Advantage and Disadvantage of In Silico Drug Discovery
Recently, in silico strategies in drug repurposing and drug discovery have been widely used due to the high cost and the time required to identify therapeutic molecules in the laboratory [189]. Computational methods aim to identify a small set of molecules with therapeutic function against the targeted protein based on the protein-ligand interactions. Moreover, computational methods enable the integration of toxicity, metabolism, and adsorption profiling to eliminate off-target molecules. It also enables identifying a single molecule against different protein targets [190]. On the other hand, some of the common pitfalls of the computational methods are the false-positive results and the essential need for combined ligand- and structure-based methods or further in vitro assays [191]. In this regard, computational methods can be divided into data modeling approaches and virtual screening. Data modeling approaches statistically determine the relationship between the structural properties and the pharmacodynamics and pharmacokinetics properties, described as Quantitative Structure-Activity Relationships (QSAR), in order to make predictions. Regarding virtual screening for SARS-CoV-2 drug discovery, protein-ligand docking is still the most structure-based method, which specifies the ligand-binding site and evaluates its affinity [192]. The virtual ligand screening approach enables the filtration process of the different libraries to identify target-specific molecules based on the predicted affinity to this biological target [192].
The in silico computational approach has made an obvious contribution to antiviral repurposing over the last decades. Interestingly, different workflows were used to develop a therapeutic drug against SARS-CoV [193]. For example, Nguyen et al. revealed the promising 3CLPro inhibitors by virtual screening of over 300 thousand molecules and obtained 53 compounds to be tested in vitro. The study resulted in the identification of two potential 3CLPro inhibitors [194]. Similar computational studies were conducted recently during the SARS-CoV-2 pandemic. For instance, Sepay and his colleagues tested the chromanone’s derivatives, showing an inhibitory effect against the MPro of SARS-CoV-2 [195].
5.6. The Pros and Cons of Wet Laboratory Research in Pandemic Era
In vitro studies enable the characterization of the viral life cycle, evolution, and infectivity. The main advantage of in vitro studies is the preliminary drug investigation and assessing the safety, toxicity, pharmacokinetics, and metabolism without exposing animal or human subjects to the risk of the experimental drugs [196]. However, most of the in vitro proved functional molecules failed in phase I clinical trials. For instance, numerous studies revealed the potential effect of remdesivir against SARS-CoV-2 when applied on Vero E6 cells in vitro [197, 198]. Conversely, different clinical studies have shown the limitations of using remdesivir since undesired side effects were observed, like the hepatic enzymes level elevation, rash, elevated bilirubin level, renal impairment, and hypotension. Also, acute kidney injury and multiple organ dysfunction were reported for some participants. In addition, other trials were not continued due to the adverse side effects in reference to the control groups [199, 200].
The third type of current study is in vivo experiments on animal models and in clinical trials. Several drugs were repurposed to act against SARS-CoV-2, such as remdesivir, favipiravir, ivermectin, lopinavir, and hydroxychloroquine, and were examined in clinical trials [201]. In other words, clinical trials were designed for the drug repurposing of FDA-approved antimalarials, HIV inhibitors, and other drugs. In contrast, most of the ongoing animal studies are done to investigate newly discovered drugs or vaccines. To illustrate, ChAdOx1 nCoV-19 is an adenovirus-vectored vaccine that encodes the S protein to stimulate the immune response. This vaccine cannot be applied in clinical trials before being investigated for the immunogenic reaction in rhesus macaques as a suitable animal model [202]. Animal models are commonly used during the pandemic as a pre-step in vaccine and drug development [203]. However, many challenges and pitfalls face researchers during conducting in vivo experiments due to the ethical considerations during the pandemic situation [204]. On the other hand, the ongoing challenges associated with clinical trials include the limited resources, patient selection, dose identification, the evolution of the viral genome, and the presence of different clades or quasispecies of virus in different populations that may affect the response to repurposed drugs or cause drug resistance [205]. For example, some factors should be considered like age, cardiovascular diseases, diabetes, and gene expression alterations. These factors should be determined in the first place since they are associated with a higher mortality rate. Therefore, the right drug and dosage need to be carefully selected for different groups of participants [205]. To sum up, the stated pros and cons of the main ongoing types of studies support the notion that in silico drug prediction and repurposing is the lowest risk with less off-target results. However, it still requires further investigation in vitro or in animal models prior to the clinical investigations.
CONCLUSION
Current treatment strategies depend on drug repurposing with careful evaluation of their efficacy in reducing viral load and relieving disease symptoms with an acceptable safety profile. Also, there are other clinical trials exploring different types of vaccines at different stages of clinical trials. In addition, bioinformatics has an important role in determining the structure, function, and origin of the virus. It could predict possible epitopes for vaccine development and help to choose the optimal animal models for in vivo experiments. Different bioinformatics networks also help determine potential drugs for repurposing based on their interaction with different viral structural units. Homology modeling has widely contributed to the prediction of the structure of some viral proteins by retrieving and aligning the sequence and comparing it with the best result of experimentally detected proteins in PDB. Also, molecular docking plays a pivotal role in the prediction of potential compounds that are likely to target SARS-CoV-2 specific structures or host cell proteins essential for viral entry and survival, guiding the design of in vitro and in vivo studies. Among different types of studies, in-silico drug design has lower risk and provides different viable information about safety and efficacy. However, it always requires further validation in vitro and in vivo on animals and humans.
CONSENT FOR PUBLICATION
Not applicable.
FUNDING
None.
CONFLICT OF INTEREST
The authors declare no conflict of interest, financial or otherwise.
ACKNOWLEDGEMENTS
The authors thank Fareed Aboul-ela for the critical evaluation of the manuscript’s English language.
SUPPLEMENTARY MATERIAL
Supplementary material is available on the publisher’s website along with the published article.