Translational Structure Map of SARS-CoV-2: A Clinical Analysis of its Origin
Abstract
Background:
SARS-CoV-2 was declared a global health emergency by WHO Emergency Committee based on growing case notification rates at Chinese and international locations. In this paper, we present an approach to understand the probable clinical origin of SARS-CoV-2.
Methods:
A combination of citation network analysis, analysis of Medical Heading Subject (MeSH) terms, and quantitative content analysis of scientific literature, was employed to map the organization of the clinical origin of SARS-CoV-2 in this paper.
Results:
According to the results of the study, a genome of the first 2019-nCoV strain in Hangzhou was obtained, and phylogenetic analysis showed the genome to be closest to the genome of a bat SARS-like coronavirus strain, RaTG13, with an identity of 96.11%.
Conclusion:
The studies show that the dead Malayan pangolins found close to the outbreak of COVID-19 in China may have carried coronavirus closely related to SARS-CoV-2.
1. INTRODUCTION
The outbreak of the novel coronavirus, SARS-CoV-2 (coronavirus disease 2019; previously 2019-nCoV), with its epicenter in Hubei Province of the People’s Republic of China, has spread to many other countries [1-8]. In January 2020, the WHO Emergency Committee declared it a global health emergency based on growing case notification rates at Chinese and international locations. The case detection rate was changing daily and it could be tracked in almost real-time on the website provided by Johns Hopkins University [9-14]; this same site showed its epicenter to be the United States of America. COVID-19 arrived in the United States in January, and as anticipated, it has dramatically increased the usage of critical care resources [14]. Three of the hardest hit cities have been Seattle, New York City, and Chicago; the documented spread of COVID-19 to Europe occurred in late January 2020, and its impact on two of the greatest affected nations (China and Italy) has then been observed [13].
SARS-CoV-2 causes acute, highly lethal pneumonia with clinical symptoms similar to those reported for SARS-CoV and MERS-CoV [8, 9]. These coronaviruses are enveloped positive sense RNA viruses ranging from 60 nm to 140 nm in diameter with spike-like projections on their surface, giving them a crown-like appearance under the electron microscope, hence the name coronavirus [12]. The SARS-CoV-2 has caused more infections, deaths, and economic disruptions than did the 2002-2003 SARS-CoV. The origin of SARS-CoV-2 remains a mystery. Bats are considered the original source of SARS-CoV-2 because a closely related coronavirus, RaTG13, has been isolated to emerge from bats [11]. The 2019-nCoV has high homology to other pathogenic coronaviruses, such as those originating from bat-related zoonosis (SARS-CoV), which caused approximately 646 deaths in China at the start of the decade. Coronaviruses are non-segmented positive-sense RNA-wrapped viruses belonging to the family Coronaviridae and the order Nidovirales, and are widely distributed in humans and other mammals [10].
A study on the genome of the first 2019-nCoV strain in Hangzhou was conducted, and the phylogenetic analysis showed the genome to be closest to the genome of a bat SARS-like coronavirus strain, RaTG13, with an identity of 96.11% [15]. However, SARS-CoV and MERS-CoV usually pass onto intermediate hosts, such as civets or camels, before transferring into humans. On 24th October 2019, Liu and his colleagues from the Guangdong Wildlife Rescue Center of China detected the existence of a SARS-CoV-like coronavirus from lung samples of two dead Malayan pangolins with a frothy liquid in their lungs and pulmonary fibrosis, and this fact was discovered close to when the COVID-19 outbreak occurred. Hence, these studies show that the dead Malayan pangolins may have carried coronavirus closely related to SARS-CoV-2 [16].
The existence of an intermediate animal host of SARS-CoV-2 between a probable bat reservoir and humans is still under investigation. The discovery of a virus closely related to the newly emerged SARS-CoV-2 in a dataset from pangolins sampled more than a year before the outbreak illustrates that the sampling of other mammals handled by humans could uncover even more closely related viruses [5].
To better understand the structure of the research on SARS-CoV-2, it is necessary to clarify first as to what are the main scientific sources and databases on SARS-CoV-2 and how they are related. Second, it needs to be determined as to how both translational and clinical knowledge on SARS-CoV-2 is interrelated to determine its possible origin. Finally, the dominant research design on SARS-CoV-2 needs to be determined according to the structure of the literature sources consulted, mainly from metadata [4].
2. MATERIALS AND METHODS
This research is based on the analysis of publicly available meta-databases on the topic, namely abstracts of scientific articles (available in the Medical Literature Analysis and Retrieval System Online MEDLINE© of the United States National Library of Medicine). We have previously developed a combination of methodologies to explore the scientific literature, which allows us to identify the main research fronts in a given field and how they are interconnected with each other. In addition, these methodologies can map the process of knowledge translation through literature networks [6]. In this study, the steps of the aforementioned methodology mentioned below were followed.
A search for research articles on SARS-CoV-2 was conducted in the US National Library of Medicine (NLM) database. Search terms were as follows: starting with title SARS-CoV-2 OR “severe acute respiratory syndrome coronavirus 2” (all fields) OR “sars cov 2” (all fields), “COVID-2019” (all fields) OR “severe acute respiratory syndrome coronavirus 2” (supplementary concept) OR “2019nCoV” (all fields) AND “coronavirus” (all fields) (database: PubMed; time period: all years). Thus, 2,037 articles were retrieved from this search.
From the total number of articles, only 84 articles related to and most relevant to the origin of SARS-CoV-2 have been selected. We have selected these 84 articles on SARS-CoV-2 due to being motivated by Bradford's Law, a method to estimate the concentration of knowledge through a small, feasible, and readable number of articles that accumulate most of the communication process through the citation network with Carrot2 software [7]. A statistical software, called R language (Aria and Cuccurullo, 2017) has been used to build a network model of citations, keywords, and bibliometric networks of the previously selected articles. R is an open-source software, which is mainly used as a platform for visualization and cluster analysis. A clustering algorithm and modularity were also used to split the model, which helped relate different research sources and, according to them, determined clear graphs of our research.
The selected articles were searched in the Carrot2 software, which is a search engine that semantically analyzes the articles for information (title and abstract) and tags them with Medical Subject Heading (MeSH) terms. A priori, we defined in clinical terms the MeSH terms that belonged to the hierarchical categories as they constituted the keywords of this research: “virus”, “infection”, “genome”, “Wuhan”, “structure”, “new coronavirus”, “COVID-19”, “2019-nCoV”, “China”, “outbreak”, “porcine deltacoronavirus”, “SARS-CoV-2” and “viral replication”. The most characteristic MeSH terms were used to label and differentiate the groups. The papers were coded according to a color, which represented a function of the clinical terms rate. The network model was displayed using the “spin glass” algorithm.
We then built a network model of inter-citation for the papers on the origin of SARS-CoV-2. The model was visualized using Gephi [3] to identify highly interconnected (dense) regions of the network model. The title and abstract of the patent families in the network model were analyzed using the semantic annotator of R. The main MeSH terms associated with the network model and dense regions were identified.
3. RESULTS AND DISCUSSION
We built different semantically analyzed network models (maps). The first map displayed the general structure and intercommunication among basic, translational, and clinical research on SARS-CoV-2, while the second map showed how the knowledge is structured and what could be the clinical origin of SARS-CoV-2. These maps have been separately described in the paper.
A citation network of 2,037 top-cited papers on SARS-CoV-2 was built (Fig. 1). Carrot2 software divided the network into six clusters (Fig. 2). These clusters of papers have been mainly organized according to different researches on SARS-CoV-2 in accordance with the MeSH terms distribution (Fig. 3). Also, a cluster of epidemiological and clinical papers has been organized with the term “COVID-19” and one cluster of papers has been focused on “animals”. The clusters were numbered according to their size rank and named with their most representative MeSH terms.
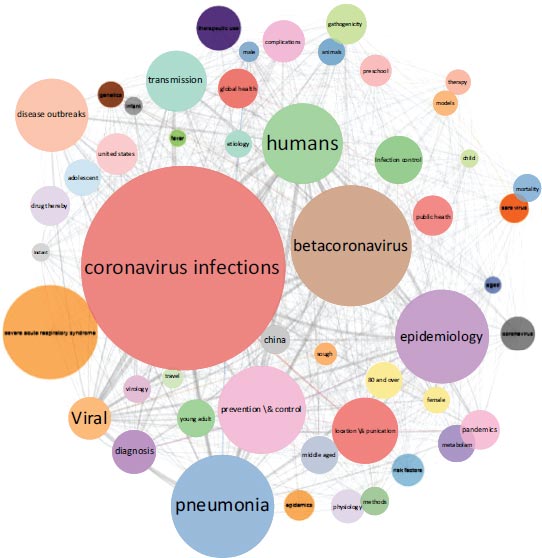
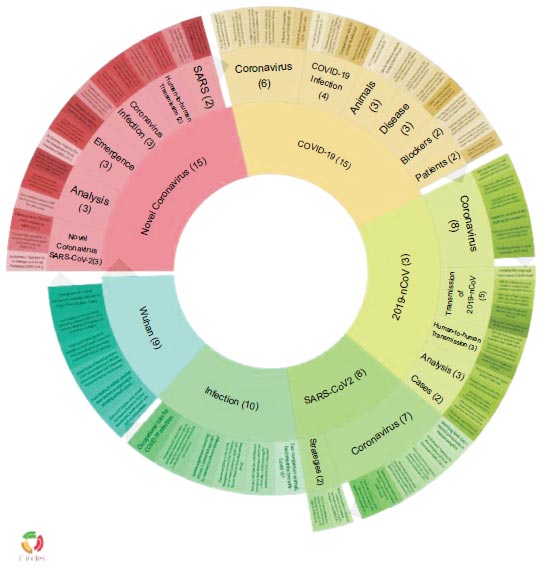
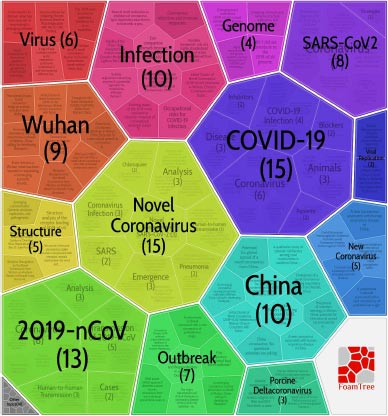
Cluster | Label | Words |
---|---|---|
1 | Antiviral agents | Drug therapy |
Pharmacology | ||
Therapeutic use | ||
Drug effects | ||
Severe acute respiratory syndrome | ||
2 | Animals | Viral |
Coronavirus infection | ||
Humans | ||
Pneumonia | ||
Epidemiology | ||
Betacoronavirus | ||
Transmission | ||
China | ||
Genetics | ||
Zoonoses | ||
Disease outbreaks | ||
Pathogenicity | ||
Chiroptera | ||
Diagnosis | ||
Methods | ||
Peptidyl-dipeptidase A | ||
Public health | ||
World Health Organization | ||
3 | Physiology | Metabolism |
Virus replication | ||
SARS virus | ||
Chemistry | ||
Antibodies | ||
Cell line | ||
Mice | ||
Virus attachment | ||
Receptors | ||
4 | Virology | Coronavirus |
Veterinary | ||
Genome | ||
Immunology | ||
Classification | ||
Viral vaccines | ||
Phylogeny | ||
Swine | ||
Swine diseases | ||
5 | Host-pathogen interactions |
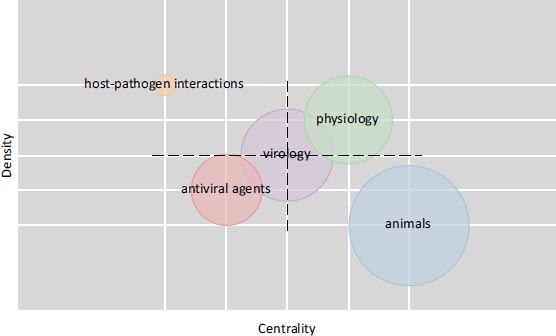
Cluster 2 consisted of 38 inter-citations. These papers exhibited the term “animal”, and were found to be closely related to the origin of SARS-CoV-2 (Table 1 and Fig. 4).
Nine of these papers were published in the Journal of Medical Virology, eight in the Emerging Microbes and Infections, and finally, seven in Nature. The paper found the most relevant has been “Probable Pangolin Origin of SARS-CoV-2 Associated with the COVID-19 Outbreak” [16]. Cluster 2 papers clearly formed a basic research front organized around the origin of SARS-CoV-2, with distinguishing terms being “viral”, “coronavirus infections”, and “humans” (Table 1).
Fig. (5) summarizes how these clusters have been organized together. Each color represents one cluster, and the arrows have been formed by the sum of the inter-citations between two clusters. To make the organization more interpretable, we hid the links formed with the origin of SARS-CoV-2.
3.1. Qualitative Content Analysis of the Network
The qualitative content analysis has been found to be consistent with the organization observed in the network of origin of SARS-CoV-2 (Fig. 5). The most distinctive term found in cluster 2 has been “animals”; however, it has not been possible to distinguish related content by network size.
The correspondence analysis plot has shown cluster 2 to involve the most content about animals and probable origin (Fig. 6). This network has been mainly connected to the rest of the citation network through cluster 2 (Fig. 5). The most distinctive words found for cluster 2 in this analysis have been “SARS-COV-2”, “coronaviruses”, “identifying”, “Malayan” and “pangolins”, and these have been found to be inter-connected.
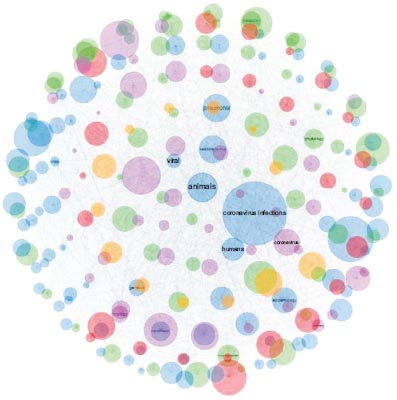
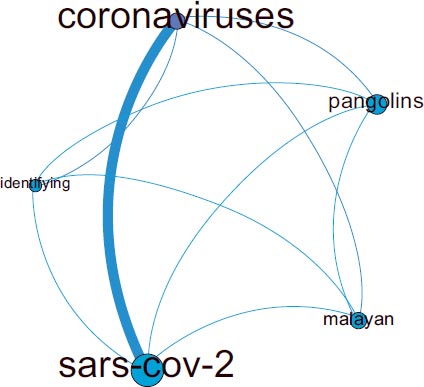
Label | Cluster | Degree | Clustering Coefficient |
---|---|---|---|
SARS-COV-2 | 2 | 65 | 0.058894232 |
Coronaviruses | 2 | 41 | 0.098170735 |
Identifying | 2 | 4 | 0.5 |
Malayan | 2 | 4 | 0.5 |
Pangolins | 2 | 4 | 0.5 |
Label | Cluster | Degree | Clustering Coefficient |
---|---|---|---|
Coronavirus | 2 | 198 | 0.030841408 |
Coronaviruses | 2 | 41 | 0.098170735 |
Outbreak | 2 | 45 | 0.128787875 |
Pneumonia | 2 | 45 | 0.135353535 |
Bat | 2 | 13 | 0.243589744 |
Discovery | 2 | 15 | 0.252380967 |
Origin | 2 | 15 | 0.27619049 |
Associated | 2 | 18 | 0.287581712 |
Probable | 2 | 12 | 0.295454532 |
Surveillance | 2 | 7 | 0.5 |
Probe | 2 | 7 | 0.5 |
Capture-based | 2 | 7 | 0.5 |
Next-generation sequencing | 2 | 7 | 0.5 |
2 | 7 | 0.5 |
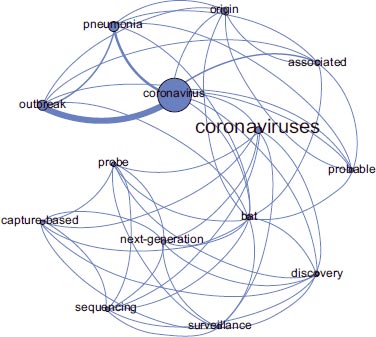
The quantitative content analysis (Table 2) suggested cluster 2 as basic clinical research focused on the probable origin of SARS-CoV-2, according to the MeSH terms distribution.
Finally, we found cluster 2 to involve the most relevant terms of “bat”, “discovery”, “origin”, “associated”, “probable” and “surveillance” (Fig. 7). Thus, cluster 2 (Table 3) and its content have been found important for our translational research focused on the clinical origin of SARS-CoV-2.
Gephi identified the origin of SARS-CoV-2 by creating smaller networks (Figs. 6 and 7).
CONCLUSION
In this paper, we have presented a semantic analysis showing pangolins and bats as likely origin of the SARS-CoV-2. First, we have searched for papers on SARS-CoV-2. Second, we have focused on the most cited documents. Therefore, in this research, we have looked for the keywords, abstracts, and titles of research sources relevant to SARS-CoV-2. We have mapped the structure and conceptual clinical origin network of SARS-CoV-2 (1). Our results have suggested SARS-CoV-2 to be originated from animals, in particular, pangolins and bats. The methodology presented in this paper may serve to map SARS-CoV, MERS-CoV, H5N1, H7N9, Ebola, and emerging SARS-CoV-2.
Therefore, given the emergence of SARS-CoV-2 pneumonia as a new infectious disease with interspecies transmission from animals, we should reflect on the origin of the human pathogens and learn from our experience. It should be taken into account that the Huanan seafood market has been trading a variety of live animals, such as hedgehog, badger, snake, and bird (turtledoves), and probably pangolin, where the first case of the COVID-19 outbreak has been reported [8, 9].
CONSENT FOR PUBLICATION
Not applicable.
STANDARDS OF REPORTING
PRISMA guideline has been followed in this paper.
FUNDING
None.
CONFLICT OF INTEREST
The authors declare no conflict of interest, financial or otherwise.
ACKNOWLEDGEMENTS
Declared none.
SUPPLEMENTARY MATERIAL
PRISMA checklist and flow diagram are available as supplementary material on the publisher’s website along with the published article.